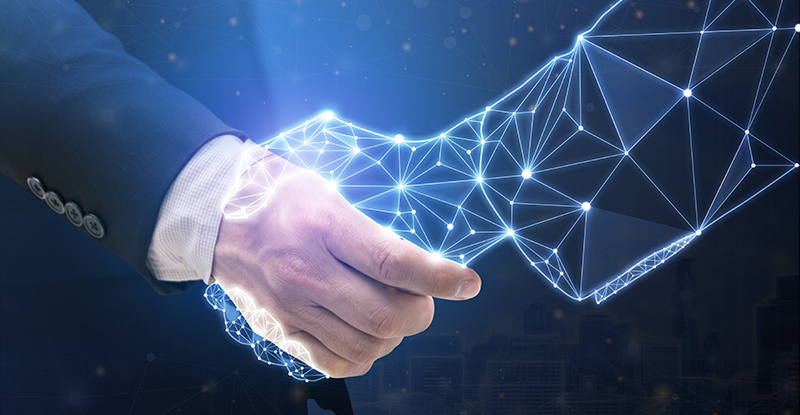
By now you’ve probably heard about ChatGPT—a chatbot1 trained with machine learning on a massive 300 billion words of data. This is an example of “generative AI”—artificial intelligence that can generate content and answer questions based on its own learning.
From successfully writing essays to creating programming code and artwork, the next levels of generative AI are tackling open and subjective problems that traditional rules-based automation simply couldn’t address. The potential power of this technology is great enough for Microsoft to start using it to revolutionize web searching and target the $150-billion search ad industry.
Models like ChatGPT will inevitably demonstrate a mix of both impressive results and obvious failures—underlining the fact that these models don’t actually understand anything (in the way humans do) and only create content based on mathematically derived patterns found in data through heavy computation. However, as finance professionals who frequently produce forecasts and projections, we know that even if predictions aren’t always (or ever?) correct, it doesn’t mean they’re not useful.
Behind the cachet of high-profile developments like ChatGPT, machine learning (frequently badged as AI) is being used increasingly—and successfully—in many areas, including financial forecasting; time series analysis; the classification of risks, customers, and debts; and behaviour mapping for marketing purposes.
But will AI/machine learning really have a profound impact on finance professionals?
Don’t believe the hype … but don’t get complacent either
Looking back at past predictions about the introduction of new technologies and drawing from personal experience working for a major research organization, I’ve seen two common patterns emerge:
- The pace of implementation is typically overestimated. Adoption generally takes longer than expected.
- The scale of the impact is often underestimated, because many secondary and later effects don’t become evident until the technology matures.
Even for simpler technologies like robotic process automation software, the pace of implementation is generally slower than predicted because implementation involves not only technology but also change management, information ownership, process engineering, and governance. Still, combining this type of process engineering with AI/machine learning is what creates the potential for “intelligent automation” that is able to go beyond simple rules-based processing and tackle more complex activities.
Although implementing these new capabilities will take time, replacing costly manual activities with increasingly sophisticated and cheaper software services and solutions make this a question of when rather than if. And even if a current role can’t be replaced entirely, that doesn’t mean it won’t be affected. If, for example, 25% of four full-time roles can be automated, these four roles can later be re-engineered into three. This is the way transformation business cases work, and future roles will generally go to those who understand and can manage these new technologies.
What role can finance professionals play?
The idea of having a data scientist on staff who can and is available to do everything (programming, statistical analysis, data visualization, and more) isn’t a scalable solution. Recognizing this, many organizations are assigning data engineering, analysis, machine learning, etc., to separate teams. Additionally, no-code and low-code tools and web-based machine-learning services are making many data analysis, visualization, and machine-learning techniques available as pre-built/configurable solutions to a much wider range of analysts and power users. And, increasingly, advanced techniques such as predictive analytics are being built into standard tools and software, like Power BI, to make them available to trained end users. So will it really be long before we see Excel provide access to machine learning in the cloud?
Certainly, for some finance professionals—for example, those working in financial planning and analysis—learning the principles and uses of some of these more advanced predictive tools and techniques is already highly valuable. Over time, however, this kind of knowledge is likely to become an expected skill.
More generally, it’s also important to understand that while there will continue to be a need for data scientists, there will be a much larger need for informed business managers and professionals who can act as intermediaries between an organization’s business requirements and its technical capabilities—what McKinsey describes as “analytics translators.”2,3 In fact, not having these kinds of intermediaries involved is one of the reasons many advanced analytics/machine learning projects fail.4
This is where you, as a CPA, can step in, once you’ve developed some understanding of advanced analytics, machine learning, and AI, and of their usefulness and applicability to your organization. Doing so will enable you to help your organization identify opportunities, build business cases, manage the implementation of new technology, and redesign processes and ways of working. Having these skills will become even more essential as a greater proportion of traditional finance and accounting activities become automated.
Seize the day
The mainstreaming of AI can either pose a challenge or provide a tremendous opportunity, depending on how well we prepare and adapt—individually, within teams, and organizationally. If financial professionals start to act sooner rather than later, I believe we can play a key role as change and transformation partners for the future.
Author bio
Simon Lindley is an international trainer, speaker, and consultant in data analytics, working with accounting institutes, training providers, and clients across the globe. He brings expertise in visual analytics, dashboard design, and predictive analytics to help clients transform traditional reporting, implement business intelligence, and develop wider analytics capabilities. Simon is a chartered accountant in the UK and a chartered engineer (IT), and he holds an MBA with a master’s diploma in corporate governance.
This article originally appeared in the May/June 2023 issue of CPABC in Focus.
Footnotes
1 A computer program that simulates human conversation. See ibm.com/topics/chatbots.
2 Nicolaus Henke, Jordan Levine, and Paul McInerney, “Analytics Translator: The New Must-Have Role,” February 1, 2018.
3 David C., “Why We Need More Analytics Translators Than Data Scientists,” December 6, 2020.
4 Dennis Ramondt, “You Don’t Have Enough Analytics Translators, Here’s Why That’s a Problem,” June 11, 2019.